The Department of Statistical Sciences and the Clinton Health Access Initiative have come together to provide an exciting opportunity for Masters students through an fellowship programme. The programme provides an opportunity for students in their dissertation year to analyse government data to answer a policy-relevant question enabling them to receive work experience that is aligned with their dissertation. MASHA facilitated the establishment of this programme and is proud to present the STA-CHAI fellowship students below.
2022 Cohort
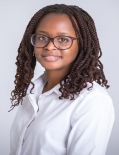
Eva Ssozi
Project title: Benchmarking Laboratory Test Ordering in Selected Health Facilities
Project description:
The cost of healthcare is a huge burden to governments across the world and the rise of laboratory test orders is a major contributor. The project investigates test ordering in government facilities in the Eastern Cape by completing a per capita analysis and the effect of electronic gate keeping on test ordering is determined. De-identified patient test data was obtained from the National Health Laboratories Service (NHLS) and evaluation of gate keeping protocols was completed in RStudio. Anomaly detection using unsupervised clustering methods was implemented to identify outlier wards, patients and facilities that are over ordering tests. The results obtained will provide a basis to make recommendations aimed at reducing unnecessary test ordering for specific tests/wards, therefore helping to reduce costs
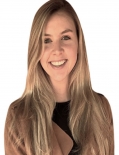
Cailin Perrie
Project title: Discrete Time Event Multilevel Survival Analysis and Machine Learning Models for Prediction and Analysis of Public Health Workforce Attrition Rates in the Eastern Cape Province of South Africa
Project description:
South Africa’s public health system budget currently accounts for an annual workforce attrition rate of 5% for health facilities in general. This rate does not consider fluctuations in annual attrition rates between cadres, across facilities, or across districts. Based on a preliminary exploratory data analysis of the EC provinces' public health HR data the annual attrition rates between 2010-2020 have consistently exceeded this budgeted 5%, with the annual attrition rate in some years reaching as high as 15.65%. The preliminary analysis further indicated that attrition rates are subject to high variation when computed at different levels (i.e. cadre, facility, district). Presently, there are no guidelines or models for predicting annual attrition within the EC public health care system, nor are there quantitative methods for identifying factors influencing attrition rates. As a result, staffing levels are determined entirely by the discretion of facility or departmental managers which, in turn, affects budgeting and impacts expenditure and resource management. Potential impact of the project include: understanding cadres/facilities/districts of high concern could ensure that employee retention efforts are directed to the places of highest priority; Enabling better policy and budget formulation with respect to staffing levels due to improved knowledge of historical and predicted future attrition rates (reducing unnecessary training/overtime expenditure and simultaneously ensuring medical care meets international quality standards).
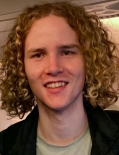
Sky Cope
Project title: An Agent-based Model to optimise Emergency Medical Services delivery in the Eastern Cape Province of South Africa
Project description:
Response time is a widely-used metric for evaluating the performance of the Emergency Medical Services (EMS) system. Recent data have shown that EMS response times in the Eastern Cape, South Africa, are typically long, especially in rural areas and urban townships. This research aims to use an agent-based simulation model to identify changes that can be made to the current allocation of EMS staff and vehicles, to provide the Eastern Cape Department of Health with a set of recommendations that can be implemented to improve patient outcomes in the Nelson Mandela Bay region.The model was trained with historical call centre data to infer the spatio-temporal distribution of EMS calls, across multiple call types and priorities between 2019 and 2021. Findings from this study will provide a basis for making evidence-based recommendations to EMS planners and personnel in the ECDoH to improve financial and patient outcomes.
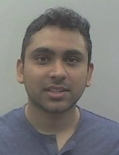
Saiheal Narayan
Project title: Case-Mix and Coding Error Detection In Western Cape Healthcare Facilities
Project description:The research aims to generate an error detection model that can flag error in diagnosis coding (ICD10) by health care staff in the Western Cape and looks at applying case mix adjustment for hospitals in Western Cape based on DRG weights from the private sector. The data looked at was on patient level and include length of stay , procedures and cost centre. Models trained to find these errors include neural networks, logistic regression, Wilcoxon signed rank test and MCA modelling. The potential of using case-mix in public sector will allow optimised resourcing and error detection model will increase data quality in the public sector.